Deep Learning: Advanced Computer Vision
This is one of the most exciting courses I’ve done and it really shows how fast and how far deep learning has come over the years. When I first started my deep learning series, I didn’t ever consider that I’d make two courses on convolutional neural networks. I think what you’ll find is that, this course is so entirely different from the previous one, you will be impressed at just how much material we have to cover.
More
Let me give you a quick rundown of what this course is all about:
We’re going to bridge the gap between the basic CNN architecture you already know and love, to modern, novel architectures such as VGG, ResNet, and Inception (named after the movie which by the way, is also great!)
We’re going to apply these to images of blood cells, and create a system that is a better medical expert than either you or I. This brings up a fascinating idea: that the doctors of the future are not humans, but robots.
In this course, you’ll see how we can turn a CNN into an object detection system, that not only classifies images but can locate each object in an image and predict its label.
You can imagine that such a task is a basic prerequisite for self-driving vehicles. (It must be able to detect cars, pedestrians, bicycles, traffic lights, etc. in real-time)
We’ll be looking at a state-of-the-art algorithm called SSD which is both faster and more accurate than its predecessors.
Another very popular computer vision task that makes use of CNNs is called neural style transfer.
This is where you take one image called the content image, and another image called the style image, and you combine these to make an entirely new image, that is as if you hired a painter to paint the content of the first image with the style of the other. Unlike a human painter, this can be done in a matter of seconds.
I will also introduce you to the now-famous GAN architecture (Generative Adversarial Networks), where you will learn some of the technology behind how neural networks are used to generate state-of-the-art, photo-realistic images.
Currently, we also implement object localization, which is an essential first step toward implementing a full object detection system.
I hope you’re excited to learn about these advanced applications of CNNs, I’ll see you in class!
AWESOME FACTS:
One of the major themes of this course is that we’re moving away from the CNN itself, to systems involving CNNs.
Instead of focusing on the detailed inner workings of CNNs (which we've already done), we'll focus on high-level building blocks. The result? Almost zero math.
Another result? No complicated low-level code such as that written in Tensorflow, Theano, or PyTorch (although some optional exercises may contain them for the very advanced students). Most of the course will be in Keras which means a lot of the tedious, repetitive stuff is written for you.
Suggested Prerequisites:
Know how to build, train, and use a CNN using some library (preferably in Python)
Understand basic theoretical concepts behind convolution and neural networks
Decent Python coding skills, preferably in data science and the Numpy Stack
- Know how to build, train, and use a CNN using some library (preferably in Python)
- Understand basic theoretical concepts behind convolution and neural networks
- Decent Python coding skills, preferably in data science and the Numpy Stack
Who this course is for:
- Students and professionals who want to take their knowledge of computer vision and deep learning to the next level
- Anyone who wants to learn about object detection algorithms like SSD and YOLO
- Anyone who wants to learn how to write code for neural style transfer
- Anyone who wants to use transfer learning
- Anyone who wants to shorten training time and build state-of-the-art computer vision nets fast
What you'll learn:
- Understand and apply transfer learning
- Understand and use state-of-the-art convolutional neural nets such as VGG, ResNet and Inception
- Understand and use object detection algorithms like SSD
- Understand and apply neural style transfer
- Understand state-of-the-art computer vision topics
- Class Activation Maps
- GANs (Generative Adversarial Networks)
- Object Localization Implementation Project
Watch Online Deep Learning: Advanced Computer Vision
# | Title | Duration |
---|---|---|
1 | Introduction | 02:36 |
2 | Outline and Perspective | 06:50 |
3 | Where to get the code | 08:27 |
4 | Anyone Can Succeed in this Course | 11:56 |
5 | What is Machine Learning? | 14:27 |
6 | Code Preparation (Classification Theory) | 16:00 |
7 | Beginner's Code Preamble | 04:39 |
8 | Classification Notebook | 08:41 |
9 | Code Preparation (Regression Theory) | 07:19 |
10 | Regression Notebook | 10:35 |
11 | The Neuron | 09:59 |
12 | How does a model "learn"? | 10:54 |
13 | Making Predictions | 06:46 |
14 | Saving and Loading a Model | 04:28 |
15 | Suggestion Box | 03:04 |
16 | Artificial Neural Networks Section Introduction | 06:01 |
17 | Forward Propagation | 09:41 |
18 | The Geometrical Picture | 09:44 |
19 | Activation Functions | 17:19 |
20 | Multiclass Classification | 08:42 |
21 | How to Represent Images | 12:37 |
22 | Code Preparation (ANN) | 12:43 |
23 | ANN for Image Classification | 08:37 |
24 | ANN for Regression | 11:06 |
25 | What is Convolution? (part 1) | 16:39 |
26 | What is Convolution? (part 2) | 05:57 |
27 | What is Convolution? (part 3) | 06:42 |
28 | Convolution on Color Images | 15:59 |
29 | CNN Architecture | 20:59 |
30 | CNN Code Preparation | 15:14 |
31 | CNN for Fashion MNIST | 06:47 |
32 | CNN for CIFAR-10 | 04:29 |
33 | Data Augmentation | 08:52 |
34 | Batch Normalization | 05:15 |
35 | Improving CIFAR-10 Results | 10:23 |
36 | VGG Section Intro | 03:05 |
37 | What's so special about VGG? | 07:01 |
38 | Transfer Learning | 08:23 |
39 | Relationship to Greedy Layer-Wise Pretraining | 02:20 |
40 | Getting the data | 02:18 |
41 | Code pt 1 | 09:24 |
42 | Code pt 2 | 03:42 |
43 | Code pt 3 | 03:28 |
44 | VGG Section Summary | 01:49 |
45 | ResNet Section Intro | 02:50 |
46 | ResNet Architecture | 12:46 |
47 | Building ResNet - Strategy | 02:26 |
48 | Uh-oh! What Happens if the Implementation Changes? | 05:17 |
49 | Building ResNet - Conv Block Details | 03:35 |
50 | Building ResNet - Conv Block Code | 06:09 |
51 | Building ResNet - Identity Block Details | 01:24 |
52 | Building ResNet - First Few Layers | 02:29 |
53 | Building ResNet - First Few Layers (Code) | 04:16 |
54 | Building ResNet - Putting it all together | 04:20 |
55 | Exercise: Apply ResNet | 01:17 |
56 | Applying ResNet | 02:40 |
57 | 1x1 Convolutions | 04:04 |
58 | Optional: Inception | 06:48 |
59 | Different sized images using the same network | 04:14 |
60 | ResNet Section Summary | 02:28 |
61 | SSD Section Intro | 05:05 |
62 | Object Localization | 06:37 |
63 | What is Object Detection? | 02:54 |
64 | How would you find an object in an image? | 08:41 |
65 | The Problem of Scale | 03:48 |
66 | The Problem of Shape | 03:53 |
67 | 2020 Update - More Fun and Excitement | 05:46 |
68 | Using Pretrained RetinaNet | 11:15 |
69 | RetinaNet with Custom Dataset (pt 1) | 04:27 |
70 | RetinaNet with Custom Dataset (pt 2) | 09:21 |
71 | RetinaNet with Custom Dataset (pt 3) | 07:06 |
72 | Optional: Intersection over Union & Non-max Suppression | 05:07 |
73 | SSD Section Summary | 02:53 |
74 | Style Transfer Section Intro | 02:53 |
75 | Style Transfer Theory | 11:24 |
76 | Optimizing the Loss | 08:03 |
77 | Code pt 1 | 07:47 |
78 | Code pt 2 | 07:14 |
79 | Code pt 3 | 03:51 |
80 | Style Transfer Section Summary | 02:22 |
81 | Class Activation Maps (Theory) | 07:10 |
82 | Class Activation Maps (Code) | 09:55 |
83 | GAN Theory | 15:52 |
84 | GAN Code | 12:11 |
85 | Localization Introduction and Outline | 13:38 |
86 | Localization Code Outline (pt 1) | 10:40 |
87 | Localization Code (pt 1) | 09:11 |
88 | Localization Code Outline (pt 2) | 04:53 |
89 | Localization Code (pt 2) | 11:04 |
90 | Localization Code Outline (pt 3) | 03:19 |
91 | Localization Code (pt 3) | 04:17 |
92 | Localization Code Outline (pt 4) | 03:20 |
93 | Localization Code (pt 4) | 02:07 |
94 | Localization Code Outline (pt 5) | 07:43 |
95 | Localization Code (pt 5) | 08:40 |
96 | Localization Code Outline (pt 6) | 07:07 |
97 | Localization Code (pt 6) | 07:38 |
98 | Localization Code Outline (pt 7) | 04:59 |
99 | Localization Code (pt 7) | 12:08 |
100 | (Review) Tensorflow Basics | 07:28 |
101 | (Review) Tensorflow Neural Network in Code | 09:44 |
102 | (Review) Keras Discussion | 06:49 |
103 | (Review) Keras Neural Network in Code | 06:38 |
104 | (Review) Keras Functional API | 04:27 |
105 | (Review) How to easily convert Keras into Tensorflow 2.0 code | 01:50 |
106 | Windows-Focused Environment Setup 2018 | 20:21 |
107 | How to install Numpy, Scipy, Matplotlib, Pandas, IPython, Theano, and TensorFlow | 17:31 |
108 | How to Code by Yourself (part 1) | 15:55 |
109 | How to Code by Yourself (part 2) | 09:24 |
110 | Proof that using Jupyter Notebook is the same as not using it | 12:30 |
111 | Python 2 vs Python 3 | 04:39 |
112 | How to Succeed in this Course (Long Version) | 10:25 |
113 | Is this for Beginners or Experts? Academic or Practical? Fast or slow-paced? | 22:05 |
114 | Machine Learning and AI Prerequisite Roadmap (pt 1) | 11:20 |
115 | Machine Learning and AI Prerequisite Roadmap (pt 2) | 16:08 |
116 | What is the Appendix? | 02:49 |
117 | BONUS: Where to get discount coupons and FREE deep learning material | 05:32 |
Similar courses to Deep Learning: Advanced Computer Vision
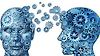
Machine Learning A-Z : Become Kaggle Masterudemy
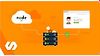
Machine Learning with JavascriptudemyStephen Grider
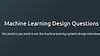
Machine Learning Design Questionsalgoexpert
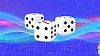
Statistics Bootcamp (with Python): Zero to Masteryzerotomastery.io
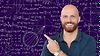
Mathematical Foundations of Machine Learningudemy
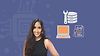
The Data Bootcamp: Transform your Data using dbt™udemy
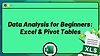
Data Analysis for Beginners: Excel & Pivot Tableszerotomastery.io
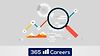
Statistics for Data Science and Business Analysisudemy
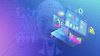
Complete Machine Learning and Data Science: Zero to Masteryudemyzerotomastery.io
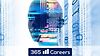